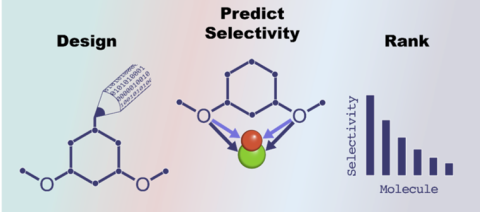
CMI researchers from Ames National Laboratory conducted the research for this highlight.
Innovation
Ligand design code and two curated datasets with AI-friendly format that quickly produce high-accuracy selectivity predictions for molecules that bind to metal ions in water.
Achievement
- Enabled for the first time fast and accurate prediction of metal-ligand binding for over 50 metal ions in water.
- Completed two well-curated and tested datasets and updated a published RE dataset, enabling machine learning.
- Provided free open-source code to enable further improvements and accessibility to the scientific community.
Significance and Impact
Prediction of metal-ion binding affinity of new ligands lays the foundation for automated design of entirely new extractant molecules for selective separations, avoiding years of trial-and-error experimentation.
Hub Target Addressed
Increasing the Speed of Discovery and Integration. Predictive Models for “Real” Materials. Workforce development.