Accelerating the discovery and design of advanced functional materials using AI/ML and exascale computing
This project develops a series of exascale-capable computational codes and workflows that integrate materials theories and methods with AI/ML tools, materials databases, as well as the software stack developed through the Exascale Computing Program (ECP). The goal is to enable dramatic speed up in the prediction of new materials in new composition-structure-property spaces, and the identification of synthetic pathways for new functional materials. The project produces AI/ML integrated and validated open-source, public-access community codes, and associated databases on exascale computing facilities.
We bring together experts with complementary skills from two national laboratories in AI/ML for material research, ab initio theory and computation, atomistic modeling/simulation of complex materials, as well as exascale computing. This team is unique and competent for addressing the basic energy sciences and exascale computing challenges. We organize our research work into two synergized thrusts: Thrust 1 - AI/ML-assisted materials discovery and design framework; Thrust 2 - Computational prediction of synthesis pathways. This project will also leverage close collaborations with experimental groups at Ames Lab to form a timely feedback loop for validation of the computational codes and predictions.
The project aligns well and supports DOE’s missions on Exascale Computing and Artificial Intelligence for Science initiatives. We demonstrate the power of AI/ML and exascale computing in significantly reducing the time-to-solution for energy and advanced technology innovations. The open-source community codes, and associated databases developed from this project will enable science-based predictive design and discovery of a wide-range of functional materials that would otherwise be impractical or impossible to investigate in a timely manner due to their complexit
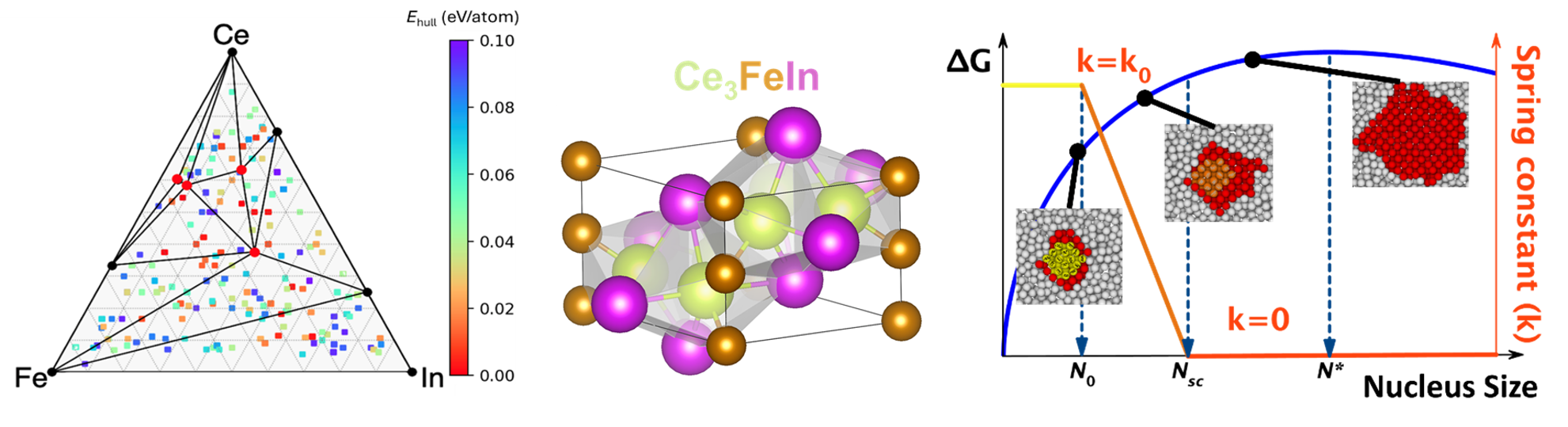