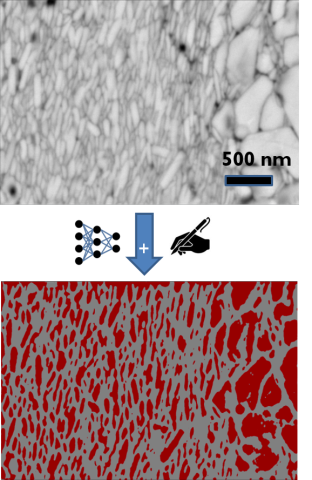
CMI researchers from Lawrence Livermore National Laboratory conducted the research for this highlight.
Innovation
An accurate microstructure segmentation software pipeline to enable automated measurement of morphology, size, and particle orientation.
Achievement
Microstructure segmentation is important for characterizing and analyzing experiments, such as for permanent magnets, where well aligned grains enable improved magnetic properties. Traditional methods require considerable expertise, human efforts; Existing deep-learning methods require lots of annotations.
Significance and Impact
This approach is adapted from the PixelPick method, a collaboration with CMU, which is easy to train—a new material class can be created within few iterations, ~10 minutes per iteration, requiring only a few training images and reduced annotation efforts. The output will quantify details of individual particles to provide ensemble statistics which can be correlated to bulk magnetic properties. This enables optimization of processing parameters to improve magnet performance.
Hub Target Addressed
Develop and deploy artificial intelligence or machine learning tools that accelerate the development of relevant technologies.