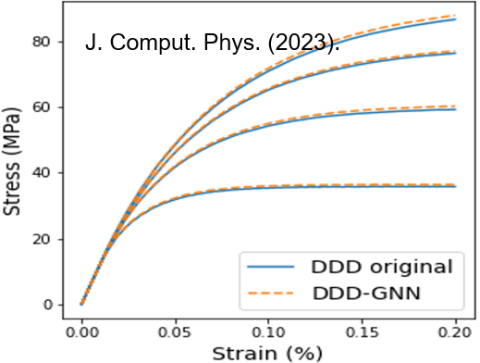
CMI researchers from Lawrence Livermore National Laboratory conducted the research for this highlight.
Innovation
Developed neural-network (NN) based surrogate models for microstructure simulation with up to 10,000X speedup
Achievement
Graphical NN models retain accuracy with much smaller computational cost in simulating dislocation motion as well as nucleation from melt
Significance and impact
- High-fidelity microstructure simulations such as molecular dynamics (MD) are very expensive.
- Faster machine-learning surrogate models allow access to much larger length- and time-scales while maintaining accuracy.
- One of the most promising approaches for modeling force fields.
Hub Level Goal Addressed
- Increasing the speed of materials discovery and deployment
- Accelerated microstructure simulation for alloys and magnets
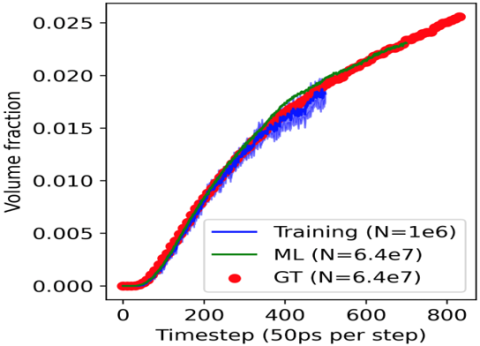