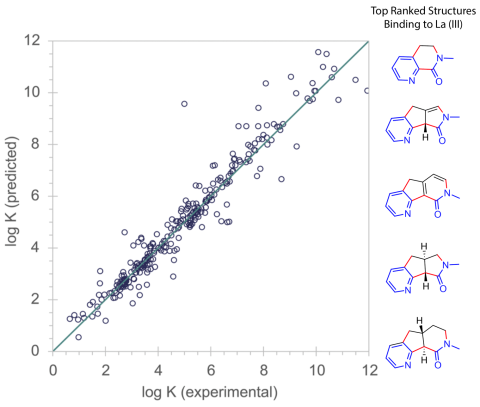
CMI researchers from Ames National Laboratory conducted the activity for this highlight
Innovation
Developed software for metal complexing ligand design from molecular fragments and machine learning-predicted binding.
Achievement
We developed and published a fast computer-aided molecular design process that leverages machine learning to predict metal-ligand binding.
Significance and Impact
- Accessible and expandable ligand design software.
- Fast, highly accurate predictions as compared to experiments or other computational methods.
- In the works: a model with greater flexibility in ligand structures through an expanded and carefully curated data set, manuscript in progress.
Hub Target Addressed
- Increasing the speed of discovery and integration
- Predictive models for “real” materials
- Workforce development