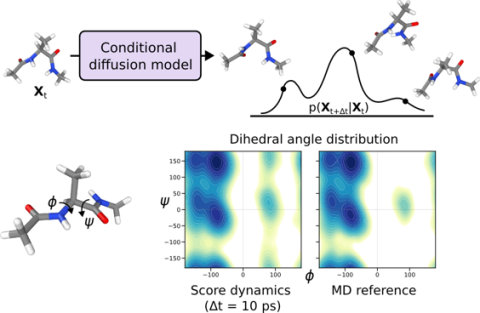
CMI researchers from Lawrence Livermore National Laboratory conducted the activity for this highlight
Achievement
Demonstrated a general framework using score dynamics (SD) to construct graph neural network models that are about 100x faster than traditional molecular dynamics.
Significance and Impact
Expanding the time and length scales addressable by molecular dynamics (MD) simulations to better understand kinetic processes, transition paths, and equilibrium distributions.
Details and Next Steps
- High-fidelity molecular dynamics are expensive and limited to 0.001 ps timesteps.
- Conditional diffusion model trained on MD data achieved 10 ps timesteps while preserving excellent accuracy.
- Future work includes quantifying uncertainty and long-term stability of SD simulations.
Hub Target Addressed
Increasing the speed of materials discovery and deployment.