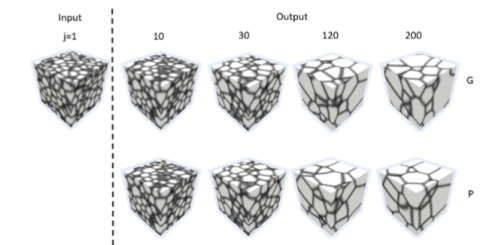
CMI researchers from Lawrence Livermore National Laboratory and Rice University conducted the activity for this highlight
Achievement
Surrogate models driven machine-learning methods have emerged as an attractive microstructure simulation tool with the potential to deliver predictive microstructure evolution dynamics with huge savings in computational costs.
Significance and Impact
This is the first Graph Neural Network (GNN) surrogate model for microstructures. Grain growth simulations are important tools to understand alloy mechanical properties and this approach accelerates simulations by ~100x.
Details and Next Steps
- Useful for both 2D and 3D simulations
- Large scale 3D simulations provide both precision and efficiency
- Grain growth simulated on both CPUs and GPUs
- Future work will generalize the models to include more anisotropic conditions
Hub Target Addressed
Increasing the speed of materials discovery and deployment.