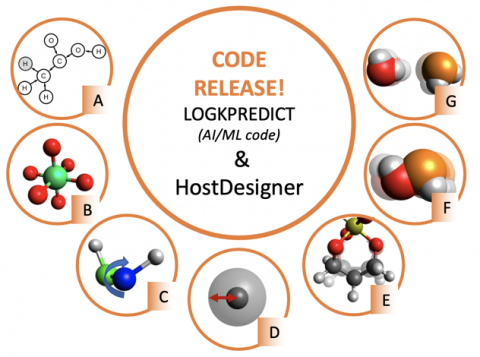
CMI researchers at Ames Laboratory conducted the research for this highlight in collaboration with the Supramolecular Design Institute
Innovation
Created AI/ML software that allows the prediction of metal-ligand complex formation constants from physico-chemical descriptors.
Achievements
Novel LOGKPREDICT code interfaced with existing structure-based design code HostDesigner to provide enhanced scoring of designed ligand architectures.
-
LOGKPREDICT, open-source software, on github.com/
- HostDesigner, open-source software, on sourceforge.net/
Significance and Impact
Designed ligands can now be rank-ordered with respect to selectivity, log(KM1/KM2), facilitating the design of improved metal ion separating agents prior to synthesis and testing.
Hub Goal Addressed
Increase speed of discovery and integration. Highly selective separation from complex sources allowing for greater supply diversification.
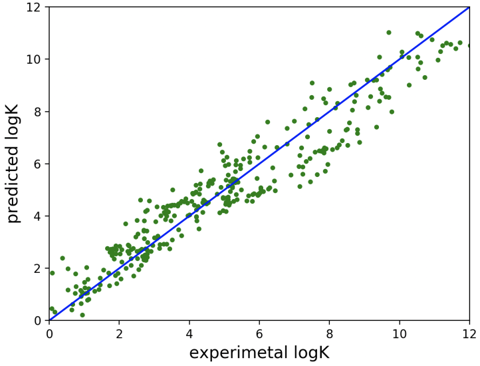